Published on the 18/01/2017 | Written by Donovan Jackson
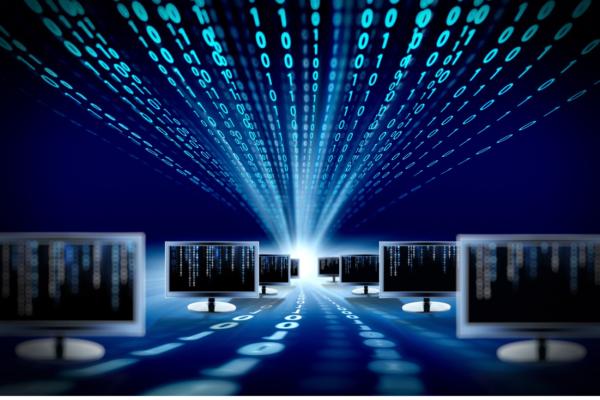
More than 40 percent of data science tasks will be automated by 2020 reckons Gartner…
Like any other discipline to which automation is introduced, more of it for data science tasks will result in increased productivity and reduced cost. Gartner, which anticipates that two fifths of the tasks data scientists perform today will be automated by the end of this decade, also said the resultant broader usage of data and analytics will drive the emergence of ‘citizen data scientists’. But while agreeing that automation is a component of the job, a local data scientist is sceptical of the concept of everyman getting in on the action. Gartner defines a citizen data scientist as a person who creates or models that use advanced diagnostic analytics or predictive and prescriptive capabilities, but whose primary job function is outside the field of statistics and analytics. At iStart, we thought of an analogy between professional and amateur photographers; both can take great pictures, but for consistently reliable results, you’d probably look to the pro. But, said Gartner in a statement, citizen data scientists can bridge the gap between mainstream self-service analytics by business users and the advanced analytics techniques of data scientists. ‘They are now able to perform sophisticated analysis that would previously have required more expertise, enabling them to deliver advanced analytics without having the skills that characterise data scientists’, it added. Andrew Peterson, data scientist at SAP solution provider Soltius, said automation is already evident in the field; for example, “One of the SAP products we license and support automates 80-90 percent of the model building process and it does that very effectively.” Which aligns neatly with what Gartner said: ‘With data science continuing to emerge as a differentiator across industries, Gartner said ‘almost every data and analytics software platform vendor’ is now focused on making simplification a top goal through the automation of various tasks, such as data integration and model building’. “Making data science products easier for citizen data scientists to use will increase vendors’ reach across the enterprise as well as help overcome the skills gap,” noted Alexander Linden, Gartner research veep. “The key to simplicity is the automation of tasks that are repetitive, manual intensive and don’t require deep data science expertise.” What isn’t automated In other words, clever people are still going to be the differentiator, something Linden confirmed; he said the increase in automation will also lead to productivity improvements for data scientists, with fewer of them required to do the same amount of work. Peterson delved further into the nuances, explaining that just running a data set through an automated algorithm doesn’t necessarily deliver useable results. “Having a detailed understanding of the models and algorithms is important. While people are working on systems that will attempt to infer the correct model from the data, the critical assumption with these systems is that the user is providing the correct data in the correct structure for the problem they are trying to solve.” Peterson said this can be ‘a dangerous assumption’; he also said Gartner’s claims around citizen data scientists are perhaps hyped. “Automation will lower the entry-level skill set somewhat, but nothing much is going to change over the next three years from a practical perspective.” “Automation will lower the entry-level skill set somewhat, but nothing much is going to change over the next three years from a practical perspective.” That goes against Gartner’s anticipation that citizen data scientists will surpass data scientists in the amount of advanced analysis produced by 2019. It said ‘a vast amount of analysis produced by citizen data scientists will feed and impact the business, creating a more pervasive analytics-driven environment, while at the same time supporting the data scientists who can shift their focus onto more complex analysis’. “Most organisations don’t have enough data scientists consistently available throughout the business, but they do have plenty of skilled information analysts that could become citizen data scientists,” said Joao Tapadinhas, Gartner research director. “Equipped with the proper tools, they can perform intricate diagnostic analysis and create models that leverage predictive or prescriptive analytics. This enables them to go beyond the analytics reach of regular business users into analytics processes with greater depth and breadth.” Picture this “It’s often subtle qualities that distinguish a stunning photo of a subject from a snapshot of the same subject; it’s reasonable to say the same about advanced analytics, with the main difference that most people are capable of differentiating between the pro and amateur photo. With advanced analytics, the inexperienced or unqualified analyst may not be aware that a distinction even exists.” In other words, said Peterson, just because you can throw a lot of data into an automated algorithm doesn’t mean you should. “But that doesn’t mean you shouldn’t, either…” … It’s time for AI to go from low impact to big bang… Mercy Radiology’s $200k cash bonus from RPA… With analytics, integration and blockchain along for the ride… Transforming inside and out is much like an episode of Grand Designs… New research suggests the human touch might be the solution to the excesses of the big data deluge…
But Peterson said the bigger story is what it doesn’t automate – and that is the translation of a business or practical problem into the appropriate type of model and data structures required to solve that problem. “It’s this translation that requires both a thorough understanding of the problem at hand, along with knowledge of the type of models or algorithms that will be required to solve the problem.”
Peterson, himself a dedicated amateur photographer, found iStart’s analogy apt. “I’d take it one step further by comparing the artistic eye and sensibilities of the successful professional photographer with the ability of the expert data scientist or analyst to interpret a problem in an analytical context. The data scientist is then able to understand what data they need and how that data must be structured before running it through any type of modelling algorithm, be it automated or not.FURTHER READING
Stuff taking AI mainstream
Switched on CEO: Dr McCann builds a bionic business
Switched on CIO: Gerard Naish on automating Ports of Auckland
Five key takeaways from St John’s digital transformation
Ethics and algorithms: Can machine learning ever be moral?