Published on the 27/07/2017 | Written by Donovan Jackson
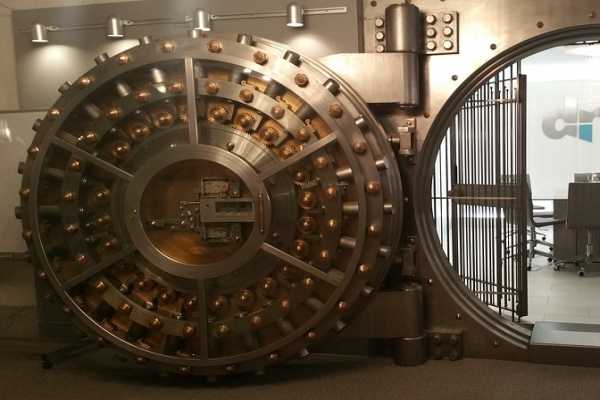
NOW Consulting adds data modelling alternative to address rapid change use cases…
With the meteoric rise of new approaches to data management, specialist information management outfit NOW Consulting (part of globally successful Kiwi data warehouse automation vendor Wherescape) is providing further options for accelerating the extraction of value from data stores. It has added the Data Vault methodology to its repertoire of solutions, describing it as is a hybrid approach which provides ‘auditability’ and offers the capability to store data from multiple sources over the course of time.
This provides for a greater level of flexibility to cope with unstructured data from the heterogeneous information systems which characterise most business environments today.
That’s according to NOW Consulting’s Data Vault lead, Chris Wyllie. “When you have multiple data sources, or need to add new sources to existing structures, it can take a lot of time to adapt traditional data warehouses to consume those data streams. Data Vault directly addresses this issue with a unique structure which makes it faster to introduce new data streams, while also providing a historical record of all the data which has come into the structure over time.”
There is also the factor that where a traditional data warehouse needs a window – generally overnight – to load data, the time required is substantially reduced with Data Vault. “Some companies would find this very attractive,” Wyllie added.
Data Vault are not new – the concept was conceived by Dan Linstedt in the 1990s, and first introduced in 2000, but only now are they being recognised as delivering real value to local businesses. The current iteration, Data Vault 2.0 is an open standard which has a focus on including new components such as big data and NoSQL.
Technical differences
Technically speaking, Wyllie explained, Data Vault modelling is designed to provide long-term historical storage of data coming in from multiple operational systems. It is also a method of looking at historical data that deals with issues such as auditing, tracing of data, load speed and resilience to change, with an emphasis on tracing where all the data in the database came from. In the Data Vault model, every row of data is accompanied by a record of its source and load date attributes.
The Data Vault difference is in how it stores the data. Traditional data warehouses most often use a ‘star’ schema, where the presentation layer is also the storage layer. Data Vault separates these layers, so that when the storage layer changes (which is a practical inevitability) there is no longer a requirement for the refactoring. “In simple terms, this means it is far faster and less costly to add new data streams to keep the data warehouse up to date, and to keep business users provisioned with all the data they need to perform analysis on to get accurate insights across the organisation,” Wyllie explained.
There’s a philosophical difference in the Data Vault approach, too. Where traditional data warehouses are fed by ‘cleansed’ data streams, a Data Vault model is built on the premise that ‘all data is relevant data’, even if it falls outside of established definitions and business rules. The Data Vault captures all data and only when reporting or extracting from the Data Vault is the data interpreted.
Practical realities of rapid change
In more practical terms, Wyllie said Data Vault is ideal for organisations that recognise the value of data, but which must cope with continual changes in the data sources. “For example, when mergers and acquisitions take place, new data streams are introduced which must be assimilated into the data warehouse if a complete picture of the organisation is to be maintained from an information perspective. A Data Vault is ideal for this scenario.”
He pointed out that change is a constant for most organisations. “The big issue is that the data warehouse is always affected by change, which can come from multiple directions. Even stable businesses have this issue, when they upgrade, swap out, or introduce new applications. When that happens, the impact on the data warehouse means a lot of refactoring in how to store and present data for users to perform their analysis.”
Horses for courses
The departure from the traditional data warehouse concepts, in which the familiar mantra of ‘garbage in garbage out’ may seem at odds with ‘all data is relevant data’, means there are structural differences in how the Data Vault is constructed.
“It requires quite a different mindset from the developers and users,” Wyllie pointed out. “Traditional models like star schema have been around forever, with proven methodologies and a large user base familiar with them. This is a new approach to the data warehouse that data warehouse teams need to invest time in to learn, but it is a better option for certain use cases – one notable example being streaming data such as you’d get from internet of things deployments, another being companies which have an explicit and ongoing acquisition strategy.”
However, he noted, for these and other use cases, the learning curve is immediately offset by the ability to rapidly introduce those new data streams, while there are ways to smooth the transition to Data Vault: “The Data Vault model is consistent and lends itself to the use of automation tools to accelerate it,” confirmed Wyllie.
“Though different, Data Vault projects don’t have to be hard and they can certainly be worthwhile.”
Pfff, Datavault is a load of hot air and completely without value. This company is just trying to hype it up to sell their consulting services.