Published on the 23/08/2018 | Written by Pat Pilcher
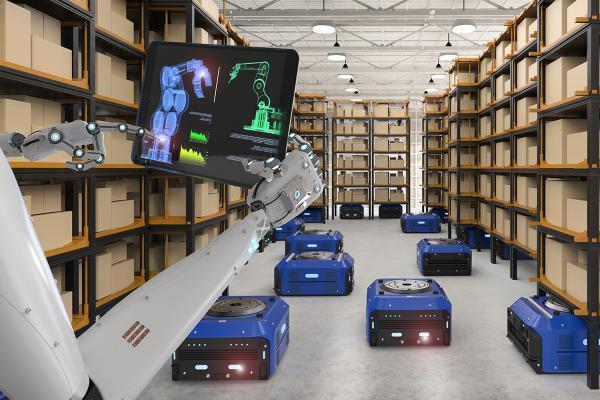
Changes are driving further demand for human workers with a digital engineer’s skill set…
We live in curious times. Technologies such as AI, machine learning and analytics are making inroads into many aspects of business, transforming how we do business and re-writing what skills are most highly desired.
A prime example of this is the accelerating rate of change happening in warehousing and supply chain logistics. The rapid change is driven by companies seeking to manage supply chain complexities and optimise performance using AI, robotics and analytics. While improved business outcomes and smoother supply chain performance are an upside, what does this mean for supply chain workers?
“The ability to discover new patterns in supply chain data on an ongoing basis offers compelling benefits.”
The increasingly global nature of commerce is creating growing levels of complexity in supply chains. Globalisation typically sees multinationals operating numerous production sites, which in turn work with multiple distribution centres, supplying thousands of end-points located around the globe.
Many are turning to a technology cocktail of AI, advanced analytics and robotics to augment existing supply chain management and reduce complexity in knowledge-intensive areas within the supply chain. These include supply chain planning, customer order management and inventory tracking.
Machine learning allows businesses to discover patterns in supply chain data using algorithms that can swiftly find which factors are most influential to a supply networks’ success. The learning process is ongoing and constant, allowing supply chain operations to respond to changing market requirements nimbly.
The ability to discover new patterns in supply chain data on an ongoing basis offers compelling benefits. There is little need for human intervention as algorithms continuously query warehouse/distribution data. Businesses can apply predictive analytics to track down key supply chain issues and opportunities with high levels of accuracy. Supply chain firms will be able to drill down into issues and optimise performance bottlenecks such as inventory, supplier quality, predictive demand forecasting, procurement/payment, production planning and transport logistics and so on.
Detractors say that the use of AI and similar technologies could see human workers become surplus to requirements, but according to Human_Machine written by Paul Daugherty and H. James Wilson, it is unlikely AI will see machines making humans redundant. Daugherty and Wilson argue that while AI can make a huge difference when used to manage high-level decision making, its real power comes to the fore when augmenting their capabilities.
In the supply chain context, Daugherty and Wilson say that humans and machines are a potent combination for roles such as supply chain planning and inventory management and identify three AI-driven roles:
The first is ‘trainers’. They will help AI systems learn. It is a broad role, and includes everything from assisting with natural language processing and translations through to teaching AI algorithms how best to mimic human behaviours.
Then there are “explainers”, people whose job will be to interpret algorithmic outputs. They will be charged with improving transparency and accountability for AI decision making processes and along with sustainers (see below), will make sure the AI/business process integration runs smoothly.
Last (but by no means least) are “sustainers”. Their job is to ensure AI systems stay on purpose and integrate smoothly with the business. They make sure that other issues such as ethics and algorithmic biases are managed, should they crop up.
The transformative outcomes achieved by teaming AI, analytics and humans are likely to be significant. A combination of machine learning data and predictive analytics plus human intuition should, in theory, make supply chain planners better placed to make more accurate forward-looking, strategic decisions. The constant ongoing machine-level focus on processes/inputs and outputs should also see AI-based systems able to spot and intervene to correct issues. The upshot of this is that managers will (in theory) spend less time on reactive problem-solving and more focusing on growing the business.
The net result is a move away from slow and inflexible supply chain models, to an increasingly dynamic and responsive AI driven supply chain model. Complex issues such as planning multiple supply chains to meet the needs of specific customer micro-segments and managing business relationships and exceptions should become significantly less complicated. From a future HR perspective, the importance of strong analytical skills looks set to only grow as demand for human workers with a digital engineer’s skill set increases.