Published on the 12/09/2018 | Written by Michael Devlin
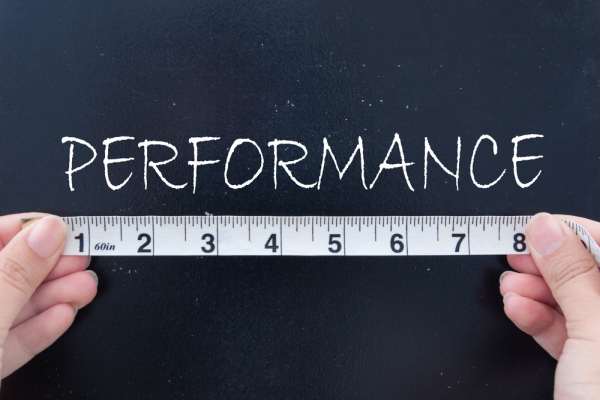
Eek! A machine is going to tell me I’m not good enough?…
We hear a lot about artificial intelligence and machine learning as if they are future concepts with no practical applications. But in the project management and business transformation space there are real-world applications of AI and ML showing very positive results. One of the challenges of assessing the effectiveness of a transformation project is measuring the effectiveness of people. ML can be applied to measuring and isolating the people characteristics of these temporary organisations that make up a project to determine if people are performing well or not. When something goes wrong in a big, complex transformation project it can be difficult to pinpoint the exact cause and effect, but it’s imperative to determine the root cause. It is quite a complex environment to even measure these problems and that is one reason why traditional project management methodologies and tools haven’t attacked the problem. “Implementation of targeted ML-generated advice enabled a complete project turn-around in a very short period of time.” We are using ML for this, as part of our AI assurance approach, and it is proving very useful. ML enables us to isolate and measure the people performance characteristics of a transformation project, that are predictive of success. With ML we can very accurately determine the probability of success, to derive improvement actions. Why machine learning? The old approach to solving the problem is to gather data, see what occurred most often and draw conclusions as to why the project failed. The problem with that method is in such complex systems trying to draw cause-and-effect it is difficult. You would need an enormous data set that is much larger than most organisations can effectively gather and process. ML can make quite accurate predictions on smaller, but higher-quality, data sets. There is a a need to train the machine learning system and we have done this via the characteristics we have isolated and measured in our challenged project recovery work. Over time we trained the ML to learn from each project what the characteristics of success look like. It has taken eight years to train, but now our ML system learns on its own and is very accurate at predicting success or failure and advising how to improve your odds. The practical outcomes? We have worked on financial services projects and one client ran our machine learning system a number of times across a recovery project. It gathered environmental data about the project and ran it back though the algorithm which correlated the characteristics of the target project with past projects. The implementation of the targeted advice generated by the ML system enabled a complete project turn-around in a very short period of time. We could then provide very specific advice on how to reform or improve the project to improve the probability of successful delivery. Transformation success or failure almost always links back to people. This is a fairly well accepted proposition – everyone knows people are a problem and how they behave impacts projects significantly. AI is the only effective way to accurately measure something as complex as behaviour in a group context. The silver bullet is finding a connection back to people being the biggest risk. For multiple parties to come together and be successful with a transformation project there needs to be good risk management. For example, a banking project will bring together a number of big suppliers and all those parties would be managing their own program risks and outcomes. One example of what we can do with ML is precisely measure how much these groups are sharing common risks. You can’t expect groups to abandon their own interests, but business leaders must have visibility to the degree of risk alignment in order to manage a successful outcome. Project and transformation success is a perennial challenge and sometimes improvement is as simple as shining a light on a problem, measuring the gap and raising the conversation at senior levels. For the first time ML is enabling this conversation. AI and ML are great examples of emerging technologies helping to improve an age-old challenge like transformation project success. I encourage you to look at how your organisation is challenged by projects and processes, and consider how a data-based solution like machine learning can be applied for better outcomes. Michael Devlin is the managing partner at Certus3, an independent IT project management company.